Talking HealthTech: 348 – Theory and reality of adopting AI into clinical care. Mark Nevin, EY Australia & Mitchell Burger Sydney Local Health District

Source: talkinghealthtech.com
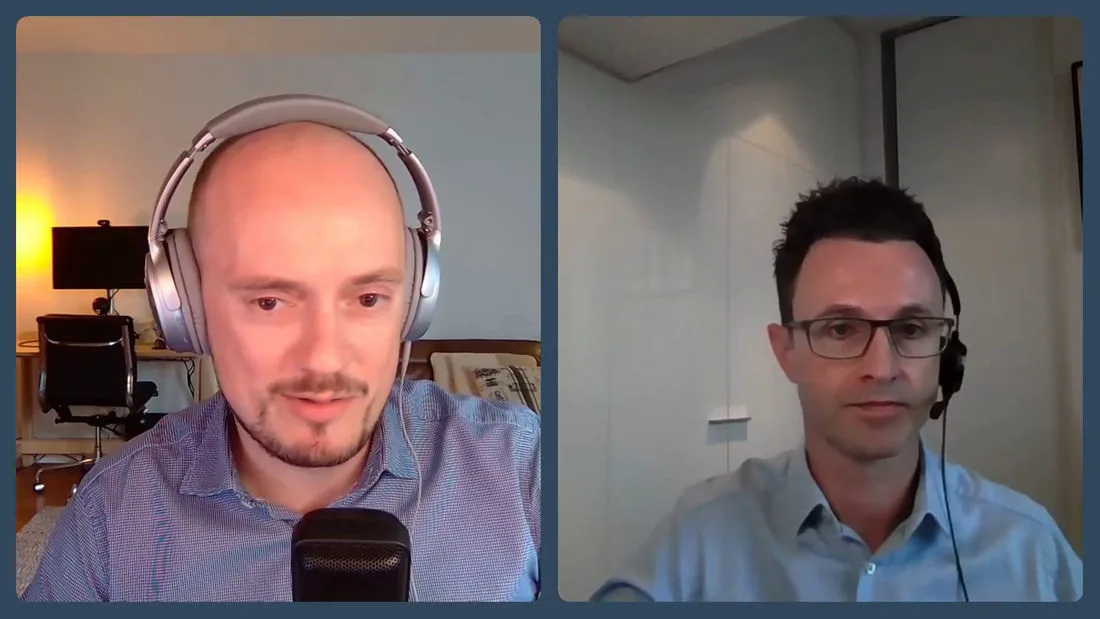
Provided by:
Talking HealthTech
Published on:
9 May 2023
This episode talks about the ethics of AI in healthcare, what standards and publications can help guide deployment, the challenges AI system designers and implementers face when putting principles and standards into practice, the role of leaders in overseeing AI deployment, workforce upskilling to work with AI, and clinical governance of AI deployment.
Meet Mark Nevin
Mark is part of the Health and Human Services leadership team in the Sydney office of EY Oceania. Mark has 25 years of experience in healthcare service provision, policy and strategy across multiple jurisdictions. He has worked mainly in sectors that rely on medical imaging, such as optometry, radiology, and oncology; AI is revolutionising all three, and he has worked in that space for the last four to five years. Mark was awarded a fellowship at AIDH in 2020 for his ground-breaking work in telehealth and the development of ethical principles, standards, and workforce upskilling in AI.Â
Meet Mitchell Burger
Mitchell is the Strategy, Architecture, Innovation and Research Director at Sydney Local Health District. He is also a Scientia PhD candidate in the School of Population Health at UNSW Sydney—researching the safe and equitable implementation of AI in public health—as well as an Adjunct Senior Lecturer in the Discipline of Biomedical Informatics and Digital Health at the University of Sydney. He holds a Master’s in Public Health from UNSW Sydney.
AI in Healthcare
AI in healthcare has been a topic for a long time, but in 2023, it’s getting to the next level. There is much attention on AI now. Multiple potential applications exist, such as medical imaging and diagnosis, hospital workflow management, payer solutions, quality and safety, medical error reduction, and precision medicine. AI is on the verge of going mainstream, which is very exciting.
Ethics of AI in Healthcare
When considering deploying complex new technologies like AI, it is important to consider ethical issues such as safety and bias and how that can influence decision-making. Additionally, given the black box-type nature of AI, complex questions can arise regarding accountability, workforce readiness, transparency of tools, and how clinicians can understand and interpret them. It will bring new players into the healthcare space, like data scientists and how they will incorporate into the team. There needs to be a solid governance framework to ensure that risks are managed systematically.
We need transparency and no black boxes when it comes to artificial intelligence in healthcare, and more and more people are being exposed to AI (e.g., playing with ChatGPT). This raises questions about whether it is appropriate for clinicians to ensure the validity of machines’ decisions and how to share accountability across decision-making systems. AI also broadens the set of people within a district or within a health service who have an account, and it will change clinical governance and insurance paradigms.
Standards in AI
Mark worked at the College of Radiologists for a number of years, and the radiology sector has been a leading light in this respect. He worked closely with the board and faculty councillors and some of the senior clinicians and senior staff to determine how the sector should respond. They looked at ethics, standards of practice, and regulation to ensure AI is regulated appropriately. Standards of practice are critical to guide deployment at a large scale, as they set industry best practices. Looking at what evidence is available and then where there’s no evidence. Consensus can develop through the process of developing those standards.Â
The key guardrails that standards can bring to the procurement of AI tools include looking at the suitability of the AI tool for a particular patient cohort, whether it has been exposed to data concerning First Nations communities, whether the findings are transparent and explainable by human experts, and whether the workforce needs to be trained and up-skill in respect of AI generally. There is also a vital governance component in relation to standards: clinical governance looks broadly at developing protective measures, safety and productive measures and determining where accountabilities lie in respect of the technology. Standards of practice can frame all of this and give them on an ongoing basis.
Trust and AI
Trust comes from understanding, but not everyone needs to understand the technical complexities of artificial intelligence and become coders—people in health need to understand standards and guardrails. There is a need for AI companies to engender trust in their technology around how it’s been ethically developed. Trust is critical regarding these new technologies, as they give us something to build. It is also crucial for health leaders to have done their due diligence in respect of the technology so that they can deploy it safely.
On the Ground in the Health District
The year 2019 has been referred to as the peak AI principles. There were thousands of ethical frameworks and best practices produced globally. The real issue for frontline health services is that the gap between technical standards, regulations, and actual practice needs to be more manageable. Frontline health services are working to build institutional competencies in this area, such as ensuring that systems are not subject to discriminatory bias through the New South Wales AI assurance framework process with the AI review board.Â
The recommendations made by the TGA for wound care were constructive and positive, and one of them was around assessing whether the technology used is suitable for the population. The question is, how much responsibility does a frontline health service have to assure a technical product that has already been through regulatory approval? Health services want to avoid having to redo everything, and they want to be able to rely on the regulatory processes and say, well, this has got approval, so we’re just going to start using it and get the benefit.
Clinical Governance and Innovation
Governance may slow down innovation and create barriers, but it can also fast-track the execution and implementation of tools. Approving these products for use is an excellent accelerator, as it allows other health services to rely on that experience and go for it. The robustness of the regulatory process is also important.
The medical device regulation aims to ascertain whether they’re safe to place on the market for sale. However, this doesn’t determine that it’s safe to be used in every context across various healthcare settings. Clinical governance becomes important around taking the fact that the tool is regulated, but is it suitable for use in this context? Standards of practice and ethical guidelines, et cetera, are abstract as well in their application, so someone needs to sit and think about how we apply that in our clinical setting. There is also an essential human element around how you use these technologies, which the regulation piece should cover.
Clinical governance is part of the puzzle. For example, research has taken a long time to assess two devices, a pulse oximeter and a temperature probe, for automated detection of deterioration for remotely monitored patients. This research has raised questions about the fitness of the data for driving automated decision-making, such as: is it valid and reliable enough to drive truly automated decision-making? Additionally, it raises the question of whether to separately conduct 12-month research projects on every device we want to use, as there are three and a half thousand devices we could be using.Â
Health services are looking to implement complex models of care that embed the use of AI, and the assurance of that stream of data is a clinical governance question. The amount of time and effort involved in the assurance process, which can take up to 12 months, is annoying and a cost for suppliers trying to get their products assured across multiple markets. There are also open questions about whether to accept extensions of the FDA approval process within Australia.
Challenges of AI Systems in the Real World
There is a level of irony in implementing AI solutions to make a process faster, which was initially a problem caused by technology. Health leaders in different districts face the challenge of stitching together manual workflows between existing IT systems. It isn’t easy to iterate up to a stage where it’s efficient and effective. There is an opportunity to use robotic process automation (RPA) a lot more in health than we currently do. Still, there is a risk of spiralling into this ever-fulfilling prophecy of technology to fix technology. Transitioning from research and innovation into production at scale is difficult, but there are ways to do it. Getting good at procurement is an excellent way to do it, and if you’re clear about what you’re doing, you can streamline the process and start small with proofs of concept and scale up. New South Wales Health has lots of support to help navigate the process, but getting used to the arcane policies takes time.
Responsibility of Leaders in AI Deployment
At the end of the day, leaders in healthcare are taking these calculated risks all of the time around the deployment of new technologies. Leaders should leverage their processes and the technical readiness of an AI tool for deployment, leveraging clinical governance capabilities and having a framework in place to manage risks. The workforce is a long-term, exciting piece of AI technology.
Health Workforce and Technology
Healthcare professionals have a duty to stay current with new technologies and treatments, processes, et cetera. As AI becomes embedded into routine practice, there is a duty and a requirement to upskill for sectors in which AI technologies are advanced. Medical specialists and medical specialist trainees have expressed the desire to upskill alongside AI, but the health workforce needs to upscale and reach a new threshold for that. This includes understanding the ins and outs of how technology is developed and the risks and oversight procedures and things they’re responsible for when making a decision based on an AI tool.
There’s a risk of automation bias, which is the tendency to rely on technology that is right most of the time. This is due to the relationship between the clinician and the patient and the service and service provider across the network. The clinician is responsible for applying AI in respect of an individual decision about a patient’s diagnosis, treatment management, whatever that might be. If something goes wrong regarding that patient, a large portion of the liability will rest with them. Still, there is also some overarching responsibility for the organisation that decided to implement technology in the first instance.
It is important to understand the concepts of shared accountability and responsibility for the different complexity components. Getting everybody on the same page upfront is essential before deploying the technology. The standards of practice developed previously look at breaking and allocating those responsibilities across the different actors involved. Ultimately, it is going to be a shared accountability piece. However, when it comes to using it with a particular patient and if something goes awry, the clinician will be responsible for the foreseeable future.
Summing Up
Health services are in the business of innovation and adoption of new technologies, and existing governance processes, frameworks, and ethical principles should be used to facilitate the adoption of AI. It is also important to build institutional competencies across the workforce and governance to understand how AI affects accountability equations.
There are quite a few complexities to wade through in respect of AI starting to get deployed at a significant scale and the whole wealth of new applications as well that are coming downstream. Trying to bridge that gap between the ethics and the standards, what will happen at the clinical coalface, and how the workforce is prepared to work safely. You need to rely on more than regulation to deploy AI safely in healthcare. Still, you have to leverage your capabilities and learn from the safe deployment that’s already happened in other parts of healthcare.